- Data cleansing and data enrichment, though distinct, are crucial processes in data management.
- Cleansing rectifies errors and inconsistencies, ensuring accuracy and reliability, while enrichment adds context and depth from external sources.
- Combination of both enhances data quality, usability, and decision-making, encouraging innovation and competitive advantage in organizations.
Data cleansing and data enrichment serve distinct purposes and functions in data management, with complementary processes that help to enhance data quality. Knowing their main differences and intricacies is crucial for efficient data processing and aggregation operations.
Table of Contents
Despite being frequently mentioned together, these two processes follow separate paths to enhancing the quality and usability of datasets. While data cleansing eliminates duplicates, inaccuracies, and outliers, data enrichment adds context and depth from additional data sources.
In this article, we have explored the complexities of data cleansing and data enrichment to help organizations apply the right techniques for specific data issues. This clarity will allow you to save resources and drive better results with optimized data quality, usability, and informed decision-making.
Data cleansing vs data enrichment
Data cleansing and data enrichment are related processes but they differ in objectives and methods. The following table illustrates how these two processes differ.
Aspect | Data Cleansing | Data Enrichment |
---|---|---|
Focus and goals | Data Cleansing Eliminating errors and inconsistencies to ensure data accuracy | Data Enrichment Appending existing data with additional information to enhance data quality, depth, and context |
Purpose | Data Cleansing Ensuring the foundational quality of data | Data Enrichment Enhancing its value and utility for various applications and business processes. |
Processes | Data Cleansing Standardization, deduplication, validation | Data Enrichment Standardization, deduplication, validation |
Techniques | Data Cleansing Data profiling, fuzzy matching, validation rules, automated tools and scripts, etc. | Data Enrichment Web scraping, API integration, Natural Language Processing (NLP), etc. |
Primary benefit | Data Cleansing Improved accuracy and reliability | Data Enrichment Enriched, contextualized data for better insights |
Challenge | Data Cleansing Ensuring that all entries are uniform and accurate | Data Enrichment Ensuring the quality and reliability of external data sources |
How are data cleansing and data enrichment used?
Data cleansing and data enrichment are employed in different scenarios to achieve high data quality.
Data cleansing corrects errors in data to ensure accuracy, completeness, and consistency. It’s crucial for areas like customer management, financial reporting, and compliance, where accurate data is vital.
Data enrichment enhances existing data with external information, adding context for better customer understanding, personalized marketing, and improved decision-making. It’s essential in market research, lead generation, and recommendation systems to reveal hidden insights and opportunities.
These processes are often sequential, with data cleansing preceding data enrichment. Data cleansing creates a reliable base for adding the information that data enrichment provides.
Data cleansing and data enrichment, while distinct, can overlap in various scenarios. For instance, during data enrichment, if external data sources contain errors or inconsistencies, we may apply data cleansing techniques to ensure data quality.
Some integrated data quality solutions merge data cleansing and enrichment into a single workflow, cleaning, augmenting, and validating data concurrently. This approach goes beyond merely rectifying errors and inconsistencies; it enhances your data with valuable external information, enriching its context and depth.
Using a comprehensive and technology-enabled approach to B2B data sourcing, validation, and enrichment, tailored specifically for a US-based client, we delivered an accurate and updated b2b database.
Enhance the quality and accuracy of your business data
Tap into the potential of data cleansing and enrichment.
Applications of data cleansing and data enrichment across industries
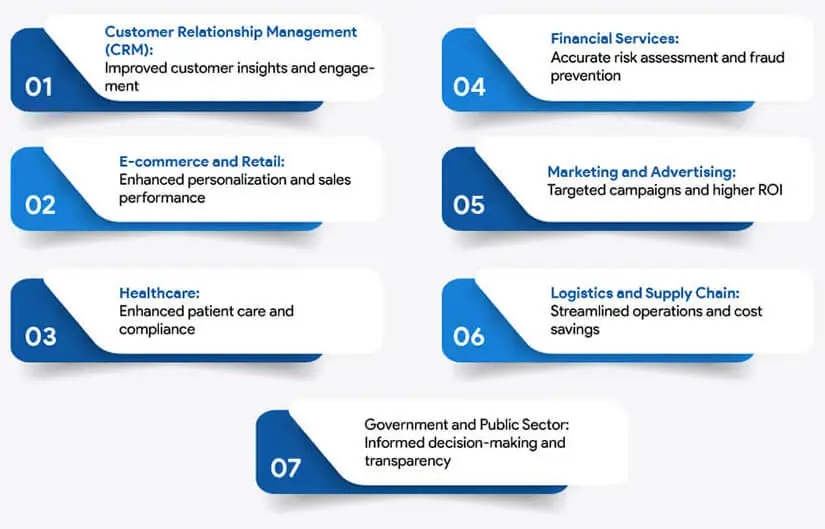
Data Cleansing Use Cases
Customer Relationship Management (CRM)
- Error correction: Identifies and rectifies errors in customer records, such as misspelled names, incorrect contact information, or duplicate entries. Also, if a CRM system has duplicate records for the same customer, data cleansing would merge them into a single, accurate profile.
- Consistency: It ensures data consistency by standardizing formats. For instance, if phone numbers are stored in various formats (e.g., (123) 456-7890, 123-456-7890, or 123.456.7890), data cleansing would normalize them to a single format for easy retrieval and analysis.
Tailored strategies empowered a trade and investment client to unlock unparalleled marketing opportunities. Validation, classification, and enrichment of customer profiles in their CRM database enhanced their marketing opportunities.
E-commerce and Retail
- Inventory management: Ensures that product information, such as names, descriptions, and pricing, is accurate and consistent across all channels. For instance, if a retailer offers the same product online and in physical stores, data cleansing ensures that the product details are consistent to avoid customer confusion.
- Order processing: Verifies shipping addresses, reducing the risk of delivery errors or returns due to incorrect addresses. For example, a customer may unintentionally input the wrong ZIP code; data cleansing corrects such errors.
- Customer communication: Ensures email lists are accurate and up to date, reducing bounce rates and improving campaign effectiveness. For instance, if a customer unsubscribes or changes their email address, data cleansing updates the database accordingly.
Healthcare
- Patient records: Data cleansing reduces medical errors by ensuring accurate patient records. Data cleansing, for instance, fixes typos in a patient’s name or date of birth, avoiding confusion throughout therapy.
- Billing and claims processing: Accurate billing and insurance claims require clean data. Data cleansing verifies patient insurance details and eliminates duplicate claims, decreasing claim denials and delays.
Financial Services
- Risk assessment: Customer data, transaction records, and credit histories are cleansed to assure accuracy. Data cleansing removes duplicate entries and outdated contact information from a bank’s client database, improving risk assessment.
- Regulatory compliance: Data cleansing ensures KYC and AML compliance by keeping accurate records. For example, if a customer’s identification information is inconsistent across different accounts, data cleansing standardizes it to meet compliance requirements.
- Fraud detection Data cleansing can identify transaction data anomalies. For example, data cleansing might prompt further investigation if a customer’s account is accessed from various places.
Marketing and Advertising
- Audience segmentation: Data cleansing ensures that consumer data is accurate and current, enabling marketers to create targeted audience segments. For instance, if a marketing database contains obsolete email addresses, data cleansing eliminates them, thereby decreasing email reject rates and enhancing deliverability.
- Personalization: Data cleansing eliminates duplicates and inconsistencies in customer records, enabling marketers to send personalized content and offers. For instance, a retailer can use cleansed data to address customers by their preferred names in email campaigns.
- Campaign analytics: To figure out how well marketing campaigns are working; you need accurate info. Cleaning up data helps keep results from being affected by mistakes in the data. For instance, if a campaign report shows click-through rates that are way too high, cleaning up the data can show if any mistakes caused the problem.
Logistics and Supply Chain
- Inventory management: Data cleansing ensures that inventory data is correct and up to date, including stock levels, item descriptions, and locations. For example, if a warehouse management system contains duplicate product entries, data cleansing consolidates them to prevent overstocking or stock-outs.
- Order processing: Data cleansing validates and standardizes shipping addresses, reducing the likelihood of delivery errors. For instance, if an order contains an incomplete address, data cleansing prompts the system to request missing information.
- Demand forecasting: Data cleansing eliminates anomalies in historical sales data caused by data entry errors. For example, if sales records contain irregularities due to typos, data cleansing corrects them, leading to more reliable forecasts.
Government and Public Sector
- Public records: Data cleansing ensures that public records are accurate and up-to-date. Data cleansing updates outdated addresses in a government database, decreasing delivery challenges for tax forms and voter registration materials.
- Fraud prevention: Data cleansing can help detect anomalies in benefit claims or tax filings. For example, if multiple individuals claim the same dependents in tax records, data cleansing can flag these discrepancies for investigation.
- Data sharing: Government agencies often need to share data across departments. Data cleansing ensures that data transferred between agencies is consistent and free of errors.
Data Enrichment Use Cases
Customer Relationship Management (CRM)
- Customer profiling: Creates more complete client profiles by adding external data to CRM. For example, if a CRM system only contains basic contact details, data enrichment can add socio-demographic data, purchase history, or social media activity, providing a deeper understanding of each customer’s preferences and behaviours.
- Personalization: Enriched data enables personalized marketing efforts. For instance, if a CRM system knows a customer’s recent purchases, it can recommend similar products or tailor promotions to their preferences.
E-commerce and Retail
- Personalization: Augments customer profiles with additional information such as browsing history, purchase behaviours, and demographic data. For example, if a customer searches for handbags frequently, data enrichment can identify this pattern and recommend relevant products when they visit the website.
- Inventory optimization: Retailers can make informed judgments about which products to stock with the aid of enriched data. For instance, by analyzing customer reviews and sentiment data, retailers identify which products are popular and which may need improvements or discontinuation.
- Competitive analysis: Data enrichment can reveal pricing, product offerings, and consumer reviews of competitors. This information allows retailers to change their strategies and remain competitive.
Healthcare
- Patient insights: Data enrichment adds external data sources like socio-economic data, social determinants of health, and lifestyle information to patient records. This helps healthcare providers gain a more holistic view of patients. For instance, if a patient’s EHR is filled out with information about what they eat and how often they work out, doctors can make more personalized care suggestions.
- Clinical decision support: Enriched data supports clinical decision-making. If a patient’s medical history includes fitness tracker data, doctors can remotely monitor activity levels and change treatment programs.
- Research and population health: Data enrichment aids in research and population health management. By combining patient data with external sources like epidemiological data, healthcare organizations can identify trends, disease outbreaks, and at-risk populations more effectively.
Check out how compilation and enrichment of 500 leads everyday enhanced the mailing list performance for a healthcare marketing and advertising company.
Financial Services
- Customer profiling: Enhances customer profiles by adding external data, such as social media activity, employment history, and financial behaviour. When a bank adds information about a customer’s recent online purchases and financial aspirations, it can provide more personalized financial advice.
- Credit scoring: Adding non-traditional data sources improves credit scoring models. Financial organizations can employ data enrichment to consider rental payment history instead of just credit history, which may benefit individuals with limited credit histories.
- Investment and portfolio management: Data enrichment can provide valuable market data, news, and economic indicators for wealth management. For instance, enrichment can assist portfolio managers in making informed investment decisions by incorporating real-time information on market trends and global events.
Verification of thousands of records with details across 80+ fields and filling up missing details enhanced revenues by winning multiple bids to supply financial data.
Marketing and Advertising
- Customer profiling: Additional data enriches consumer profiles for improved understanding and targeting. For instance, if a marketing database only has basic personal information, data enrichment can add details about customers’ interests, hobbies, or social media use to make content and suggestions more relevant to them.
- Lead scoring: Enriched data facilitates the identification of high-quality prospects by revealing their engagement and behaviour. For instance, if a B2B company enriches its leads with information on company size and industry, it can more effectively prioritize leads based on their conversion potential.
- Competitive analysis: Data enrichment can provide valuable information about competitors and market trends. By incorporating news and social media mentions into market research data, advertisers can gain insight into consumer sentiment and emerging trends.
Logistics and Supply Chain
- Supplier information: External data regarding suppliers, such as financial stability, prior performance, and geopolitical considerations, are added through data enrichment. For example, by enriching supplier profiles with data on their creditworthiness, supply chain managers can make informed decisions about supplier relationships.
- Route optimization: Enriched data includes real-time traffic, weather, and road condition information. By enriching route data with live traffic updates, logistics companies can optimize delivery routes to avoid delays and reduce fuel costs. Route optimization for a global waste tyre management company improved order pick-up efficiency by 50%.
- Inventory visibility: Data enrichment enhances inventory visibility by incorporating data from sensors, RFID tags, and IoT devices. Data enrichment can give real-time temperature and humidity data for perishable items in cold chain logistics, ensuring quality.
Government and Public Sector
- Citizen services: Data enrichment enhances citizen profiles with additional information, such as demographics, socioeconomic data, and preferences. For example, when a government agency enriches citizen data with information about preferred communication channels, it can tailor notifications and alerts accordingly.
- Emergency response: Enriched data can be invaluable in emergency response scenarios. Emergency responders can target more efficiently if 911 call data includes real-time location and medical history.
- Policy making: Data enrichment can provide insights into public sentiment and emerging trends. By enriching social media data with sentiment analysis, government agencies can gauge public opinions on policies and initiatives, helping in informed decision-making.
6 best practices for data cleansing and data enrichment
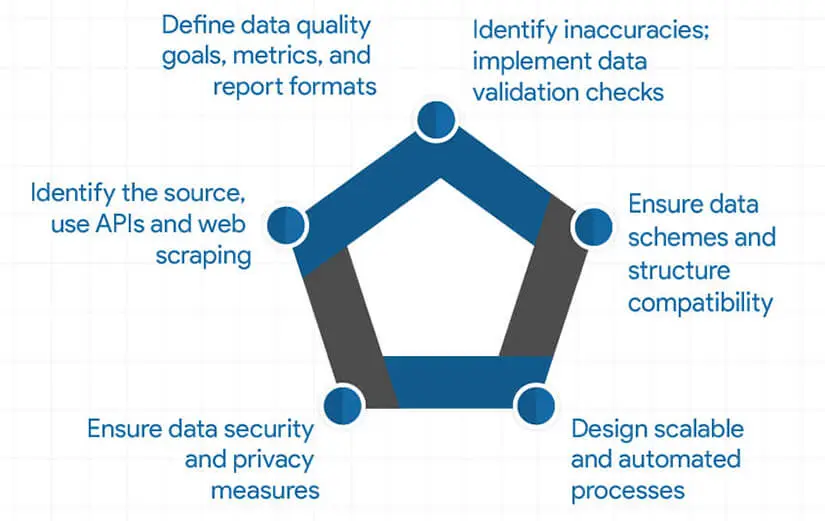
Want to unlock the full power of your business data!
Leverage the synergy of our data cleansing and enrichment processes.
Conclusion
Understanding the distinctions between data cleansing and data enrichment is essential for effective data management. Data cleansing serves as the bedrock, rectifying errors, inconsistencies, and redundancies to ensure data accuracy, reliability, and compliance. It lays the groundwork for decision-making and operational efficiency.
In contrast, data enrichment supplements the cleansed data with external information, enhancing its depth and context. This process accelerates the usefulness of datasets, uncovering hidden insights, fostering better customer understanding, and facilitating personalized experiences.
The synergy between data cleansing and enrichment is evident; the former is a prerequisite for the latter. Together, they empower organizations to extract the full potential of their data, fostering innovation, gaining a competitive edge, and elevating customer satisfaction.